Statistical Process Control
The statistical process control (SPC) is comprehensively used in manufacturing environments and it is an important global trend. There is wide use of statistical Process Control in industries to monitor the process parameters. Statistical Process Control is a process for open or closed loop control of manufacturing processes based on statistical methods. This procedure assists in monitoring the process behaviour. It is a standard method for observing and controlling processes on the basis of measurements of arbitrarily selected samples. Bulk of management studies have demonstrated that Statistical Process Control is an analytical decision making tool which permits firms to observe when a process is working accurately and when it is not. Variation is present in any process, deciding when the variation is natural and when it needs correction is the key to quality control. Statistical process control maintains definite standards without inspecting and regulating every step and product of the process. SPC is utilized in manufacturing and service applications. It allows a series of steps planned to create a constant and reliable result, or process, to remain autonomous from stable oversight. SPC can be used in such varied environments as chemical processes or information management.
Management literature revealed that the process of statistical process control was originated by Walter A. Shewhart at Bell Laboratories in the decade of1920s. Shewhart developed the control chart and the notion of a state of statistical control in 1924. Statistical control is equal to the concept of exchangeability evolved by logician William Ernest Johnson in the same year. Shewhart conferred with Colonel Leslie E. Simon in the application of control charts to munitions manufacture at the Army's Picatinney Arsenal in 1934. That triumphant application helped to persuade Army Ordnance to engage AT&T's George Edwards to consult on the use of statistical quality control among its divisions and contractors at the outbreak of World War II. W. Edwards Deming requested Shewhart to talk at the Graduate School of the U.S. Department of Agriculture. Deming was famous architect of the quality control short courses that trained American industry in the new techniques during WWII. W. A. Shewhart identified that a process can contain two types of variation. Variation contributes to random causes and/or to assignable causes. Later on, Deming developed the terms of common cause variation (variation due to random causes) and special cause variation (variation due to assignable causes). Common cause variation is an intrinsic part of every process. Usually the effect of this kind of variation is less and results from the normal rhythm of the process. Special cause variation is not an inherent part of the process instead this kind of variation emphasizes something unusual taking place within the process and is created by factors that were not part of the process' design. However, these causes are exchangeable and in most cases can be removed.
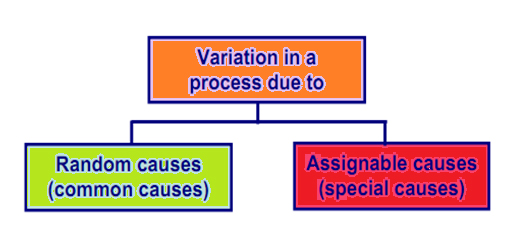
Theoretical studies denoted that SPC combines thorough time series analysis with graphical presentation of data, and can provide early insights into the data in a manner understandable to a wide range of audiences (Benneyan, Lloyd, and Plsek, 2003). According to Thor and colleagues (2007), SPC can be "an influential and versatile tool for managing changes in healthcare through quality improvement.
The techniques of Statistical Process Control when applied to measurement data, can be used to emphasize areas that would benefit from more analysis. These techniques allow the user to identify variation within their process. Good understanding this variation is important for quality improvement.
Statistical Process Control Charts are basically simple graphical tools that facilitate process performance monitoring, intended to identify which type of variation exists within the process, designed to underline areas that may involve further investigation, easy to make and interpret. The main goal of Statistical Process Control is to guarantee that the designed process output is achieved and the connected customer requirements are satisfied. In this process control, arbitrarily some parts are taken from the manufacturing process and then their characteristics are measured and revealed on the control charts. Statistical indicators are determined from the measurements and used to assess the current status of the process. If required, the process is rectified with some appropriate actions. There are two types of SPC tools used in industries which include the run chart and the control chart. They are easy to create and there is no need of specialist software. In Run Chart, a time ordered sequence of data, with a centreline drawn horizontally through the chart. A run chart facilitates the monitoring of the process level and identification of the type of variation in the process over time. The centreline of a run chart consists of either the mean or median. The mean is used in most cases unless the data is discrete.
Major Steps to create a Run Chart
- Preferably, there should be a minimum of 15 data points.
- Draw a horizontal line (the x-axis), and label it with the unit of time.
- Draw a vertical line (the y-axis), and scale it to cover the current data, plus sufficient room to accommodate future data points. Label it with the outcome.
- Plot the data on the graph in time order and join adjacent points with a solid line.
- Calculate the mean or median of the data (the centreline) and draw this on the graph.
There are numerous techniques of Statistical Process Control that can be applied to data. The trouble-free SPC techniques to put into practice are the run and control charts. The main purpose of these techniques is to categorize when the process is displaying unusual behaviour. In Control Chart, a time ordered sequence of data, with a centreline calculated by the mean. Control charts bring the addition of control limits (and warning limits optional). A control chart enables the monitoring of the process level and identification of the type of variation in the process over time with additional rules associated with the control (and warning) limits. Control charts are a vital tool of constant quality control. These charts demonstrate the discrepancy in a measurement during the time period that the process is observed. Control charts check processes to show how the process is performing and how the process and capabilities are affected by changes to the process. This information is then used to make quality improvements. Control charts are also used to verify the capability of the process. They can assist identify special or assignable causes for factors that obstruct peak performance. Control charts explain if a process is in control or out of control. They illustrate the variance of the output of a process over time, such as a measurement of width, length or temperature. Control charts evaluate this variance against upper and lower limits to see if it fits within the expected, specific, predictable and normal variation levels. If so, the process is considered in control and the variance between measurements is considered normal random variation that is inherent in the process. If, however, the variance falls outside the limits, or has a run of non-natural points, the process is considered out of control.
Major Steps to create a Control Chart
- Select the most appropriate control chart for data, which is dependent on the properties of your data.
- Proceed as for the run chart, using the mean as the centreline.
- Calculate the standard deviation (sd) of the sample using the formula listed in the appendix (for appropriate chosen control chart).
- Calculate the control limit.
- Calculate the warning limits (optional).
Statistical Process Control Charts are typically plotted over time for a single process. Nevertheless, it is also possible to construct SPC Charts at a static point in time for a process carried out by manifold institutions. Companies all over the world have adopted the Shewhart chart as their main SPC method. Though the Shewhart chart provide basic tool for statistical process control applications, its assumptions are challenged by many contemporary manufacturing environments. For instance, when standard control limits are used in applications where the process is sampled frequently, autocorrelation in the measurements can result in out-of-control signals.
Benefit of Statistical Process Control over other control methods is that production is less affected. There are less disruption and process delays than with more direct assessment and inspection methods. The important variable in maintaining Statistical Process Control work environment is determining the needed statistical sampling. Constant sampling of every aspect of the process defeats the purpose, but statistical analysis with long sampling intervals can allow discrepancies and poor quality results to slip through. When executing Statistical Process Control system, the sampling should at least signify usual quality issues already established. SPC relies heavily on control charts, continually improving the statistical analysis, and experiments planned to create possible variations for additional statistical results. Control charts track the various causes and solutions for statistical variations. The control chart results and ongoing experiments give the essential information for constantly improving the statistical model for the process.
SPC techniques provide early information on process performance, and may be used to measure the need for a summative evaluation before such an appraisal is undertaken. This could be especially useful for processes that are recognized to contribute to positive outcomes, or where processes are valued in and of themselves. SPC methods shun lack of power issues from small sample sizes. In many efforts to bring about system change, some outcomes are best measured at the system level but only a small number of practices may participate. This sample size issue makes other kinds of evaluation challenging because of lack of statistical power (Peikes, Dale, Lundquist, et al., 2011). SPC uses the power of frequent measurement to provide information on whether real transformation is occurring. This tactic also determines unusual events. Statistical Process Control can also be used to follow occurrence of unfavourable events that tend to be rare, such as failure to address a test result. Using a special type of control chart (a g-chart, where g symbolizes geometric distribution), evaluators can follow the days between events to provide a visual representation of the current status and progress for avoiding rare events (Wall, et, al, 2005). Statistical Process Control shortens interpretation of data to avoid wrong conclusions. Visual depiction of change using SPC tools is particularly helpful for some stakeholders who may not prioritise statistical significance when drawing conclusions about an involvement. SPC provides a graphical option to other methods that aids assessment of whether the pre- and post-intervention means of a variable represent stable measurements. If either measurement is not constant, assessors and stakeholders can be alerted that the difference in the two means may not represent real change (Carey, 2003).
Disadvantage of Statistical Process Control Implementation
Statistical Process Control can take time to apply thoroughly but applications do show that there are few disadvantages to SPC. Its application must remain appropriate and useful, rather than becoming a system 'for its own sake'. Problems can occur in introducing it to affirmed innumerate. SPC needs smart application and expert consultation. Thor, Lundberg, Ask, et al. pointed out that "SPC cannot solve all problems and must be applied wisely. There are many opportunities to go wrong" (2007). Thor, Lundberg, Ask, et al. also raised the problem of autocorrelation in repeated measurements on the same subject (2007). This is a big problem in more frequent measurement, such as hourly.
Steps in Statistical Process Control
In order to implement appropriate Statistical Process Control it is imperative to do good planning and data collection. Statistical analysis on the wrong or incorrect data is waste. The analysis must be proper for the data collected.
Plan: Identify the problem and the possible causes.
Do make changes designed to correct or improve the situation. Study the effect of these changes on the situation. This is where control charts are used and they show the effects of changes on a process over time. Evaluate the results and then replicate the change or abandon it and try something different.
Act: If the result is unbeaten, standardize the changes and then work on further improvements or the next prioritised problem. If the result is not yet successful, search other ways to change the process or identify different causes for the problem. Control charting is one of a number of steps involved in Statistical Process Control. The steps include discovery, analysis, prioritization, clarification, and then charting. Before using Statit QC software, appropriate data must be collected for analysis.
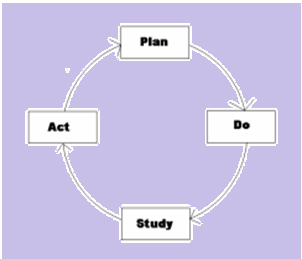
Specific SPC Procedures
The preliminary stages of Statistical Process Control involve several steps using a number of different tools. The indispensable tools for the discovery process are: Check Sheet, Cause-and-Effect Sheet, Flow Chart, Pareto Chart, Scatter Diagram, Probability Plot, Histogram, Control Charts, and Brainstorming.
Applications of Statistical Process Control: The application of SPC involves three main sets of activities:
- The first step is to understand the process. This is achieved by business process mapping.
- The second is measuring the sources of variation assisted by the use of control charts.
- The third is eliminating assignable (special) sources of variation.
It can be used in various industries for improving the quality of the product and helps in lowering the product costs as it provides a better product and/or service. The use of Statistical Process Control to software processes is a major challenge for engineers. In the manufacturing field, it is not hard to work out the relationship between product quality and the corresponding production process. But in case of software products, it is difficult to distinguish the relationship between single software process and the quality of the related software product. The major problem is that there is an important time gap between the software development process and the time of the software is being used. When testing software, it is not easy to assess which kind of behaviour the potential users will perceive as strange, and thus rate as a defect. During tests, researchers only can compare with specifications or requirements, and unwanted behaviour detected in reviews or tests are called bugs, and usually they try to remove bugs before they can be delivered to customers, who will eventually see them as defects. Another problem is quite difficult to understand the defect in software. Though there are many problems, but it is possible to apply Statistical Process Control to software processes.
To summarize, Statistical Process Control is an effective mechanism for monitoring and controlling variation in processes such as manufacturing of goods, testing, or statistical results. Dr W. Edwards Deming originated this term in 1920 who claimed the majority of variation was due to operator over-adjustment. SPC is founded on the standard that a process will reveal reliable results unless it is performed inconsistently. Statistical Process Control requires an organization to establish the process parameters that need to be monitored, create a control chart to verify the process is under control, and collect data to evaluate with the control chart to identify process variation.